
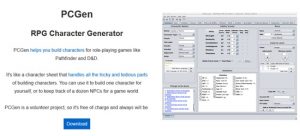
The results of the Max–Max Kurtosis Origin Distance based K-Means (MKODKM) algorithm were compared to the state-of-the-art initialization strategy on thirteen microarray genomic datasets utilizing internal, external and statistical evaluation criteria. This study presents the Max–Max Kurtosis Origin Distance (MKOD) initialization algorithm for big data multi-dimensional clustering in a single machine environment employing kurtosis coefficient and minimum origin Euclidean distance to address the initial centroid-related issue. The initial centroid is influenced by the randomization and dimension curse because it affects the effectiveness, efficiency and local optima of the cluster. The use of high heterogeneous gene dimensions reduces clustering performance and raises computation costs in initial centroid recognition. During clustering, each gene dimension is processed because each dimension describes distinct biological information. Therefore, non-stable clustering results diminish the trustworthiness of clustering results for cancer tissue identification and diagnosis. The inclusion of randomization in the initialization step produces different clustering results. The KM algorithm confronts randomization and dimensionality-related challenges during microarray genomic clustering. The KM clustering identifies hidden patterns, evolutionary relationships, unknown functions and trends in genes for cancer tissue detection, disease diagnosis and biological analysis. Microarray Genomic Data Clustering is a multi-dimensional big data application that analyzes genomic data by K-Means (KM) algorithm without any extraneous information. According to the statistical analysis, the proposed MKMDKM algorithm has achieved statistical significance by employing the Friedman test and the post hoc test. The experimental results reveal that the MKMDKM algorithm minimizes iterations, distance computation, data comparison, local optima, resource consumption, and improves cluster performance, effectiveness and efficiency with stable convergence and results as compared to other algorithms. The performance of the presented algorithm has been compared against KM, KM + +, ADV, MKM, Mean-KM, NFD, K-MAM, NRKM2, FMNN and MuKM algorithms using internal and external effectiveness evaluation criteria with efficiency assessment on sixteen genomic datasets. The MKMD algorithm enhances the effectiveness and efficiency of the KM algorithm by measuring the distance between data points of the minimum–maximum kurtosis dimension and their mean. To address this issue, this study has presented the Min–Max Kurtosis Mean Distance (MKMD) algorithm for big data clustering in a single machine environment. Therefore, the KM algorithm produces the lowest quality cluster and maximizes the computation time and resource consumption. The existing initial centroid initialization approach traps local optima due to randomization and achieves high computational cost due to the enormous interrelated dimension. The KM algorithm is highly dependent on the initial centroid because it determines the effectiveness, efficiency, computing resources, and local optima of the KM clustering. Genomic clustering is a big data application that uses the K-means (KM) clustering approach to discover hidden patterns and trends in genes for disease diagnosis, biological analysis, and tissue detection.
